FCA Market Abuse Surveillance TechSprint – What We Learned
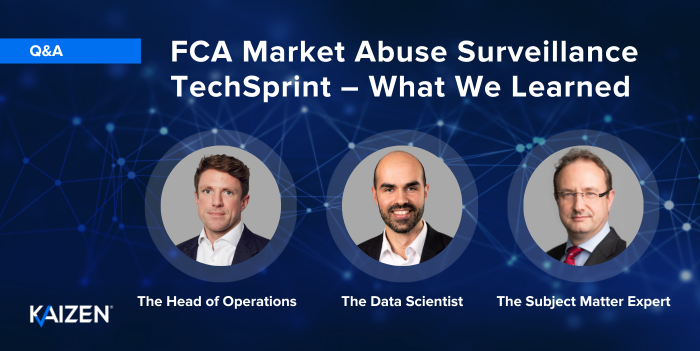
Last month we were excited to take part in the FCA’s Market Abuse Surveillance TechSprint which brought together industry participants to explore AI-powered solutions to help detect forms of market abuse.
We asked our Head of Operations for Surveillance Charles Dickson, Data Scientist Josian Quintana-Arroyo, and our subject matter expert Simon Appleton about their experiences taking part in the event and what they learned from it.
First things first, what was your role in the TechSprint?
Charles: I was the project manager, coordinating within our team and with the FCA. I ensured our project remained true to the TechSprint objectives whilst providing tangible benefits to our clients. This was done by constantly linking our research back to (i) the presentation and knowledge we would be able to share on the demo day at the FCA, and (ii) the enhancements we could roll out to our clients upon project completion.
Jos: My role was technical: data science and machine learning. I investigated the data, engineered variables and applied AI methods to detect market abuse.
Simon: I provided subject matter expertise covering market abuse, surveillance, MiFIR transaction reporting data and order book data.
What was the problem we were given to explore? Were we successful with our approach?
Charles: We chose to explore how to ‘transform market abuse surveillance by incorporating anomaly detection’. This is because we had an existing research project in this area which would allow us to immediately start applying these ideas to the vast datasets which the FCA helpfully gave TechSprint participants access to. In our case, given Kaizen’s rich history and deep knowledge in transaction reporting, it was that dataset we were most interested in.
Jos: There was success showing our approach was correct although further work is being carried out. This is because detecting market abuse is a complex process that requires a lot of analysis and consensus. There were some false positives. The variables we engineered were relevant and our approach showed results consistent with other datasets which is how we knew we were on the right track. However, many of the potential anomalies flagged required further investigation. This ‘further investigation’ aspect of product development is a critical part of developing a meaningful algorithm.
Simon: It was clear that our data scientists, programmers and product development staff benefitted from having access to an extensive and broad dataset covering transaction reports, orders and prices. We also further advanced our understanding and usage of AI in the anomaly detection process.
What was your biggest learning from taking part in the TechSprint? Do you have any advice for individuals and firms taking part in future events?
Charles: Thanks to AI, we have a rapidly improving toolset available to us. It is our responsibility to apply these with careful thought and discretion so that the resulting output is explainable and defensible, both for clients and regulators. With the vast options available, it is best to have a targeted approach to return specific answers rather than generating an exponentially growing set of questions. To get these answers implemented with clients, you need to prove additive value to existing rules-based monitoring – reduced false positives with better true positive detection, allowing compliance officers to concentrate more of their time investigating breaches and near-misses.
Jos: We learned a lot from engineering a model with so much data. We also had the chance to refine our current algorithms as all the fresh data allowed us to shed light on our own work: for example, we reconsidered the importance of two variables that we currently use. Having to explain our findings to an audience also made me think of the problem from a different point of view and I am now testing the same models with a simpler approach which will make them more easily interpretable.
My advice to others would be to move fast and come up with end-to-end solutions and improve them later, time feels a lot shorter when having to handle so much data. Also to be honest with yourself about the results if they are not as good as expected, there is a lot to learn from what doesn’t work as intended.
Simon: Although obvious, the importance of having data scientists, programmers, product and market abuse surveillance experts working well together and learning from each other made it exciting and resulted in a positive atmosphere and outcome. Selecting people with the relevant, complementary skills, knowledge and a collaborative approach would be my main suggestion.
Along with our TechSprint participation, we recently announced we are introducing a new AI powered voice transcription functionality on our communications surveillance platform. What is your advice for firms when assessing AI powered tools?
Charles: Firms should run AI powered tools in parallel with conventional processes to ensure they are achieving at least the same effectiveness before migrating fully to AI. This would involve a multi-month period with in-depth comparison and human feedback. Categories for assessment would include transparency, defensibility, effectiveness, efficiency, and synergies to other monitoring processes.
Jos: Some tools are very easy but expensive to implement especially when scaling up. Some more rudimentary approaches are effective albeit more difficult to implement, and they will be worth the saving in costs. Be realistic and well informed about what the latest technology can and cannot do. Some tools can improve performance even if they don’t do the work for you; they could still be valuable.
Simon: Given that this is a fast-moving environment, keeping your options open, opting for shorter contract periods and selecting a provider that has hedged their bets, rather than opting for a single solution.
- To learn more about the FCA TechSprint and how we are implementing AI into our integrated surveillance platform, please get in touch.
- For background on Kaizen’s approach to Generative AI, read our blog from earlier this year – Unleashing AI to Transform Regulatory Compliance.